Introduction
In the realm of science fiction studies, despite the recognition of Mary Shelley’s Frankenstein as a seminal work authored by a woman, the genre has predominantly been shaped by male writers, both in terms of influential texts and the number of contributors. This male dominance was largely unchallenged until the 1960s and 1970s New Wave movement, which saw the rise of female authors like Ursula K. Le Guin, Doris Lessing, and Margaret Atwood, introducing a fresh, gender-diverse narrative to the field. Similarly, in China, the presence of female science fiction authors offering their unique perspectives is a relatively recent phenomenon, as noted by Song (2022, p. 126), who suggests that the significant contribution of these writers has only become apparent in the last decade of the 21st century.
Another perspective argues that Chinese science fiction, unlike its Western counterpart, has long lacked a gender consciousness. This is primarily because science fiction in China has predominantly existed within the realm of science popularization, rather than fully embracing its literary attributes. As a result, it has carried a certain national mission, which has been “unrelated to gender” (Yao et al., 2021, p. 349).
However, in recent years, with the emergence of numerous anthologies of female science fiction, such as She: Classic Works by Chinese Women Science Fiction Writers, The Way Spring Arrives, and the Her Science Fiction series, there has been a significant increase in domestic attention towards the group of female science fiction writers.
A common perspective in the field of literary studies regarding women’s creative endeavors suggests that their inherent sensibility empowers them to craft narratives with richer emotional depth, delicately and keenly portraying the intricate web of emotions between characters. As Chen Jirong aptly states (2022, p. 94), “Emotive storytelling, owing to its nuances and delicate cognitive experiences, constitutes a notable characteristic of women’s literature.” Wu Yan also comments on clusters of female science fiction writers, analyzing their works and stating (2011, p. 70), “Asimov did write about love, about emotions, but these affections, lacking the complexity we have just discussed … Female writers, on the other hand, are completely different. Their love and hate intertwine.”
Wang Siyuan, in her analysis of recent anthologies with female science fiction authors, notes the emphasis on feminine characteristics, such as the “romantic and poetic” elements in their novels (2022, p. 26). She contrasts Liao Shubo’s “The Cartographer” with Liu Weijia’s “The Town Under the Tower”, pointing out the distinct emotional focus in each. Liao’s work vividly details a romantic storyline, while Liu’s narrative centers on the town’s defense mechanism, illustrating the gendered approach to emotional content in similar thematic contexts.
Even some female science fiction writers themselves acknowledge the significance of emotional storytelling in their works. For example, Wang Kanyu humorously coins her writings as “science fiction romance”, believing that “love can be excellently combined with science fiction creativity”. Furthermore, even in works where female authors employ a male perspective, they cannot conceal the sensibility stemming from their own female identity. Cheng Jingbo comments on Zhao Haihong’s “My Darling Darling, I Love You”, stating, “Although the story is told from a male perspective, the maternal tenderness between the lines betrays the author’s gender.” (Yao et al., 2021, p. 357).
Several quantitative studies do reveal differences in literary styles between male and female authors, as evidenced by statistical metrics such as the frequency of function words and parts of speech. Utilizing these metrics, machine learning algorithms designed to classify author gender automatically have demonstrated accuracy levels reaching 80% (Koppel et al., 2002). Nevertheless, the question of whether an author’s gender significantly influences emotional narratives remains a topic of debate. One study, grounded in autobiographical narratives, suggests that female authors tend to express more effect, connection, and factual elaboration compared to their male counterparts (Grysman et al., 2016). However, an analysis of an extensive literary corpus reveals that, while female authors historically produced a higher proportion of emotionally charged texts across 116 countries prior to the 21st century, this gender disparity in emotional expression has notably converged since the year 2000 (Lettieri et al., 2023).
Considering the varying themes and paradigms of writing across genres, it is imperative to examine gender differences in emotional narratives within each genre independently. This study will focus on a quantitative analysis of science fiction to discern any gender-based patterns in emotional expression. Science fiction is notable for its low percentage of women writers compared to men, which could accentuate the unique nature of women’s emotional narratives or assimilate them into a style consistent with the majority of works, a phenomenon that merits further exploration.
Methods
Affective computing, which involves the combination of various computational devices and algorithms to automatically identify, understand, and compute features related to human emotions, has become a research hotspot in cognitive science. Text-based emotional computing has also been widely applied in fields such as social media and e-commerce platforms. Conducting sentiment analysis on the massive textual data generated on these platforms can help governments and businesses conveniently grasp public opinion trends and emotional changes towards specific events or products, leading to significant social and economic benefits. As a result, it has received considerable attention from researchers and has become an important branch of the current field of natural language processing.
In recent years, scholars have integrated emotional computing methods into the field of literature, thereby introducing a new dimension of quantitative literary research (Kim and Klinger, 2021). Despite the prolonged history of metric literature studies, initial attempts emerging in the late 19th century (Mendenhall, 1887), the analytical focus has predominantly rested on linguistic models, regrettably neglecting the significant dimension of emotion. In contrast, within the realm of interpretive-critical analyses, emotional examination of texts has proven to be a valuable research avenue. Notably, in narratology, emotion is recognized as an intimately linked element with narrative structure, prompting the formation of “affective narratology”, an interdisciplinary narrative theory. Consequently, the advent of emotional computing can be viewed as a pivotal development bridging a crucial gap in metric literature research, while also facilitating the construction of a more advanced computational critical theory that transcends traditional linguistic models.
These methods can be classified into two main categories: straightforward approaches utilizing sentiment dictionaries or bag-of-words models, and algorithms such as machine learning or deep learning to create effective predictive models. The latter, in most cases, requires supervised training and relies on extensively pre-annotated corpus data.
In commercial applications and linguistic research, textual data annotation often takes advantage of crowdsourcing platforms, where numerous third-party annotators collaborate to complete annotation tasks. However, for corpus annotation in literary research, the qualifications of annotators tend to be higher, making it challenging to delegate tasks to ordinary individuals. For instance, in a corpus specifically designed for sentiment analysis in literary texts, the annotators are linguistics postgraduate students who engage in regular discussions with experts to address annotation-related issues (Kim and Klinger, 2018). The annotation process is time-consuming, tedious, and demanding due to the highly diverse nature of emotional expressions, the potential usage of metaphorical language in fiction, and the general interconnectedness of preceding and subsequent text. In some cases, the annotators are not ideally aligned with each other (Schmidt et al., 2018). The multitude of interpretations regarding the emotions conveyed in the text underscores its subjective nature, introducing an additional layer of complexity to the annotation process.
In contrast to computational linguistics, researchers in the field of literary computation adopt a different approach by focusing on specific works or collections of works to address particular problems. For instance, a study examining the computation and classification of emotions in the poetry of Francisco de Quevedo, a Spanish writer and poet, utilized four groups of emotion-evoking words in English (Barros et al., 2013). By utilizing an “English-Spanish” translation dictionary, a substantial number of words associated with these four types of emotions were obtained. To calculate the frequencies, the researchers simply searched for these words in the text of the corresponding poems, converting each poem into a four-dimensional array. While the lexicon employed in this study was limited and constructed for this specific task, it proved sufficiently effective for the literary computing project at hand.
Considering the absence of sentiment-annotated corpora for literary texts, the majority of current studies focused on sentiment analysis in literary works continue to rely on sentiment lexicons. This approach, as opposed to the alternative method that relies on extensively annotated corpora, offers several advantages. Firstly, it is simpler and more feasible, allowing for greater flexibility in its application. Additionally, this lexicon-based approach enables a more transparent and interpretable algorithmic framework, thereby allowing sufficient room for literary interpretation and criticism to take place alongside computational analyses.
When it comes to sentiment analysis of Chinese text, several widely used sentiment dictionaries come into play. These dictionaries include the Hownet Sentiment Dictionary, the BosonNLP Sentiment Dictionary, the Chinese Polarity Sentiment Dictionary created by the Natural Language Laboratory of National Taiwan University, and the Chinese Sentiment Vocabulary Ontology Library (CSVOL) constructed by the Dalian University of Technology. For the purpose of this study, we utilized CSVOL as the foundation for emotion computation. This lexicon encompasses over 20,000 distinct words or colloquial expressions, each annotated with its corresponding emotion polarity and emotion classification. Refer to Table 1 for further details:

The table provides information on the part of speech, emotional classification, polarity, and intensity for each word. As an illustration, the word “脏乱(dirty)” is classified as an adjective (adj), with an effective classification of NN (derogatory) and a polarity of 2 (pejorative). The lexicon categorizes sentiment intensity into five grades: 1, 3, 5, 7, and 9, where 9 represents the highest intensity and 1 indicates the lowest. In this case, “脏乱” corresponds to an intensity of 7, indicating a strong emotional impact among pejorative words. It is important to note that the lexicon further divides polarity into 0 (neutral) and 3 (both positive and negative), in addition to 1 (positive) and 2 (negative). The table also differentiates words with multiple meanings, providing sentiment values for each distinct meaning. For example, the word “好事(good deeds)” has a positive connotation with an intensity of 5 in one sense, but a negative connotation with the same intensity in another sense.
The emotions in this lexicon are classified based on Paul Ekman’s theory of basic emotions (Ekman, 1992). According to this theory, certain emotions are considered more “basic” compared to others. Ekman, along with other researchers, established specific criteria to differentiate basic emotions from other emotions. As a result, the dictionary follows a classification that includes six basic emotions: anger, fear, sadness, enjoyment, disgust and surprise. While adhering to this general classification, the compilers of the dictionary made a refinement within the “enjoyment” category. They divided it into “pleasure” and “goodness”, expanding the classification to include seven emotional categories: pleasure, goodness, anger, sadness, fear, disgust, and surprise. Each of these categories is further subdivided into 21 subcategories, as represented by abbreviations such as PH, PA, and NC in the table (Xu et al., 2008).
The process of emotional computation using lexicons is straightforward and transparent. Initially, the original text is segmented by a commonly used segmentation program “Jieba”, as there is no innate word boundary in Chinese. Next, emotion words within the text are extracted and tallied, and subsequently matched with the lexicon to determine the polarity, intensity, or classification of each word’s emotion. Finally, algebraic operations such as addition and subtraction are performed based on the corresponding values. The specific formula employed is as follows:
where S(M,k) represents the sentiment value corresponding to emotion k in part M, w denotes the emotion word identified within the text, and Pw signifies the sentiment intensity associated with this word. Additionally, NM indicates the length of the text corresponding to part M. The division of the summation term by the text length aims to facilitate comparisons between texts of varying lengths.
Another prevalent method for sentiment analysis involves classifying emotions into positive and negative categories (Anderson and McMaster, 1982). By subtracting the positive sentiment value from the negative sentiment value within a given text, a polarized value can be obtained. In this paper, we also calculate the polarity sentiment value using the following formula:
When w belongs to the positive category of emotions, i = 0, and when w belongs to the negative category of emotions, i = 1.
The traditional perspectives on the characteristics of women’s emotional narratives, as outlined in the “Introduction” section, can be broadly categorized into two levels. One pertains to the belief that women’s works often encompass a broader range of emotional elements including love, affection, friendship, jealousy, hatred, and more. These emotional elements are intricately woven into the narrative, consistently evolving alongside the storyline. The second relates to the perception that women’s works exhibit a greater degree of delicacy and sensitivity in their portrayal of emotions. Sensitivity implies a propensity for emotional fickleness, wherein the characters’ emotional states undergo significant fluctuations as the narrative progresses. Consequently, the emotional state of the characters in these works often experiences pronounced shifts throughout the story.
To quantitatively describe these qualities, we constructed two characteristic quantities based on emotional arcs. These quantities aim to capture the richness and interconnectivity of emotional elements as well as the variability and fluctuations in the characters’ emotional states throughout the narrative.
Firstly, we simply add up the emotional values of different sequences and moods of a piece to characterize the emotional richness of the piece as a whole, using the following formula:
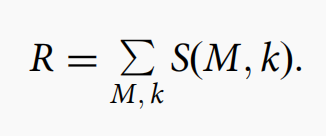
In essence, it provides a general description of the quantity and intensity of emotional words present in the stories. A higher richness indicates a greater number of emotional words from different general categories in the text, along with higher emotional intensity, suggesting that the author has adopted a more direct and impassioned approach to expressing emotions in their creation. Conversely, lower richness implies a more calm and restrained emotional narrative by the author.
It is important to note that the definition of “Richness” used here differs from the previously mentioned emotional polarity. In the latter, the values of positive and negative emotions partially cancel each other out in the calculation, potentially resulting in a low final value for texts that express complex emotional entanglements, such as love-hate relationships. In addition, the difficulty of the emotion attribution process may lead to the mixing of different characters’ emotional states with each other in the calculation, resulting in mediocre results. Therefore, in the process of calculating Richness, the intensity values of all emotions are aggregated in a positive state in order to avoid the cancellation of values between different emotions and characters, thus maximizing the extraction of all emotions present in the text.
Richness describes the story as a whole. As a result of the summation process, we lose information about the distribution of emotion values in each section, making it impossible to track and assess how emotion changes as the narrative progresses. To quantitatively measure the extent of emotional change within a work, we have introduced a novel characteristic quantity termed “Twistiness”. We can anticipate that as a story character experiences significant emotional fluctuations throughout the narrative, there will be noticeable variations in emotional intensity between adjacent sections of the text. Portraying an emotionally sensitive character or employing a subtle style of emotional writing can lead to shifts in the character’s emotions as the narrative unfolds, consequently producing marked emotional differences between neighboring sections. By aggregating these variances, we can derive a quantitative measure of the subtlety of emotional writing. Specifically, this metric is computed by taking the absolute difference between emotional values of adjacent sequences and subsequently summing them. The calculation formula is:

Put simply, an emotional arc with greater fluctuation results in a higher degree of Twistiness, whereas a smaller Twistiness indicates a smoother emotional arc.
The approach we have adopted for calculating Twistiness at a fixed total number of sequences may not be the most ideal method, because different works exhibit varying degrees of shifts in emotional tone, and the distribution of these shifts within the text is not uniform. However, it is nearly impractical to precisely divide text chunks based on the actual position and number of emotional shifts using unsupervised algorithms. Such an endeavor would require a substantial amount of manual labeling, resulting in an unacceptable level of difficulty and workload. Hence, despite its limitations, our method represents a pragmatic compromise that allows for the quantitative assessment of emotional changes in a wide range of texts.
In summary, Richness defines the author’s emotional narrative features primarily at the level of textual choices, while Twistiness is mainly concerned with the construction of the storyline and characterization. Combined, these two indicators reflect the author’s emotional narrative style from different perspectives, enabling us to quantitatively compare different authors and works within a cohesive coordinate system.
Corpus
To broaden the scope of this study, our first step is to construct a corpus comprising the works of various science fiction writers. For the selection of women’s works, we utilize the book She—A Collection of Classic Works by Chinese Women Science Fiction Writers, edited by sci-fi writer Cheng Jingbo, as the foundation. We include all the stories contained within this anthology to establish a digital corpus. The book encompasses short stories from 33 female science fiction writers, with works published between 1985 and 2021. It includes almost all notable female science fiction writers in contemporary China, thus representing the general characteristics of female science fiction.
To create a contrastive corpus of male works, we handpicked 33 male science fiction writers from different eras and selected a representative short work from each of them. To ensure structural comparability between the two corpora, we made two adjustments in the selection of male works. Firstly, we sought to align the distribution of male works across generations as closely as possible to that of female works. For example, the female works corpus consists of eight works published before 2010, with an approximate selection of two to four works for each year between 2014 and 2020. Therefore, we endeavored to maintain a similar chronological distribution structure in the selection of male works. Secondly, we aimed to ensure that male works were approximately equal in length to female works. Considering that the average length of the female works chosen for this book was around 10,000 words, we attempted to select male works of similar length or pair them with different lengths to maintain an average text length around this value. Based on these principles, we had to make certain trade-offs considering the factors of classicism, age, and length of the texts, ultimately selecting the most suitable works. Finally, the selected works are shown in Table 2.
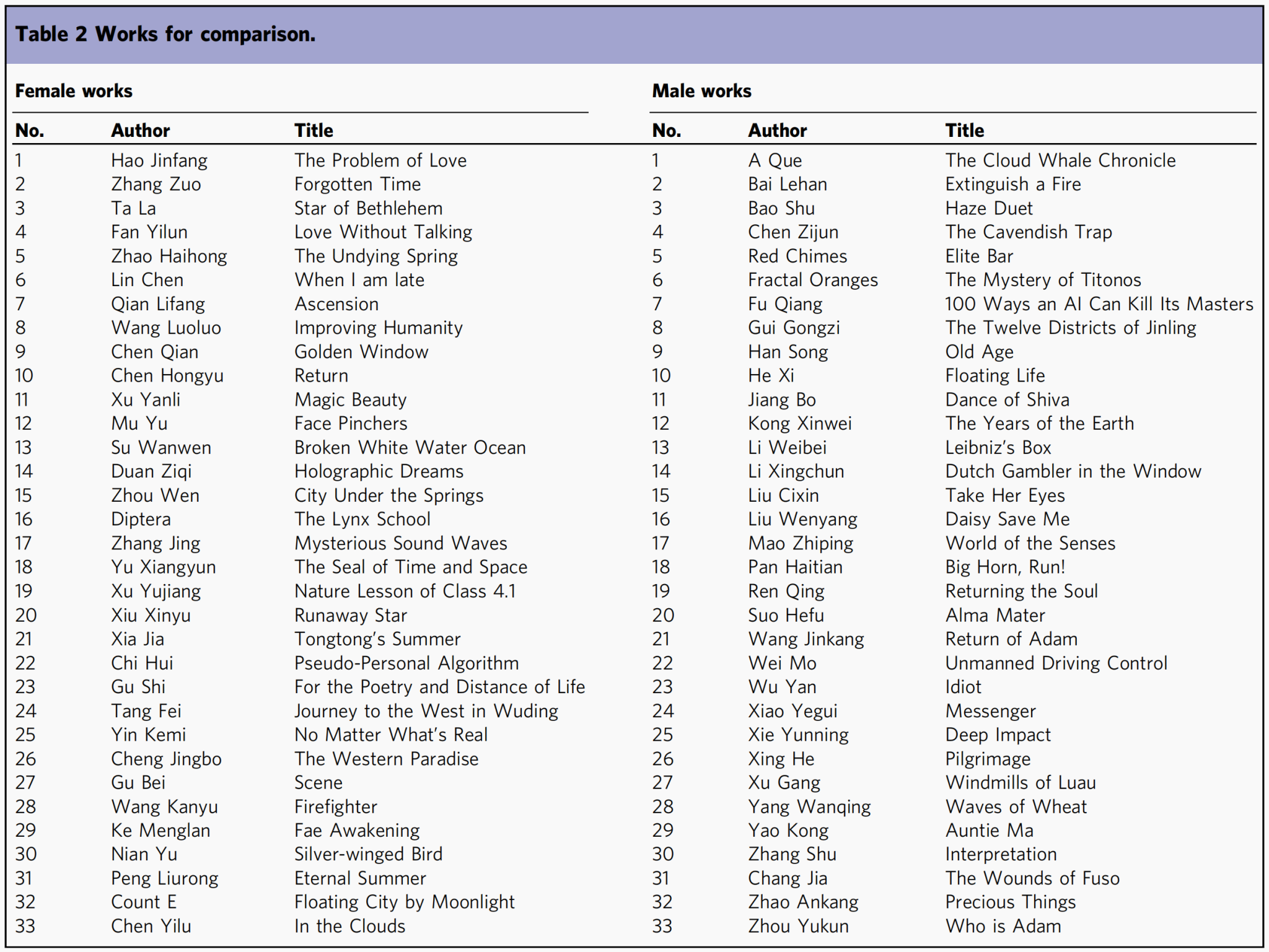
While the number of included writers was substantial in this corpus, each writer was represented by only one work. However, it is important to note that different writers may vary in terms of the number of published works and their influence in the field. Ideally, according to the principle of statistical sampling, the number of works by each writer in the corpus should be proportional to the weight of their publications. This would mean that writers with a larger number of publications should have a greater representation in the corpus in order to accurately reflect the overall structure of the current Chinese science fiction field. However, achieving this in practice is challenging.
To address this limitation, we have developed a corpus that focuses on key writers. This corpus includes 12 highly influential science fiction writers, with an equal distribution of 6 male and 6 female writers. We collected short stories ranging from 3000 to 20,000 words, aiming to include as many of their works as possible, while also avoiding comparisons between texts with significant length differences (e.g., a short story and a novel). As a result, we have obtained a larger volume corpus compared to the previous one, which is illustrated in Table 3.

Results
Emotional arcs. The concept of emotional arcs is frequently employed in the analysis of literary works, particularly regarding emotional analyses. It involves dividing the text into distinct segments, calculating the emotional values for each segment, and then examining the overall trend of these emotional values. One notable study by Reagan et al. involved mapping and categorizing the emotional arcs of 1327 stories from the Project Gutenberg fiction collection (2016). Through their analysis, they identified six core arc patterns that represent common emotional trajectories within narratives. Other studies have also utilized emotional arcs to detect storylines (Elsner, 2015), conduct narrative analysis (Elkins and Chun, 2019), and facilitate textual comparisons (Samothrakis and Fasli, 2015). Emotional arcs offer a valuable framework for understanding the emotional trajectory and structure of literary works.
Our approach is also rooted in emotional arcs, but rather than solely analyzing and categorizing the arcs as a whole, we developed new feature quantities based on the data from the arcs to obtain more in-depth information. We divided each story into five equally sized text sections based on the word count and then calculated the emotional values for each of these five parts individually, encompassing seven emotions along with their polarized emotional values. Given that our focus is on short stories, each text section had a length of approximately 2000 words, which we deemed an appropriate window width for analysis. Utilizing this methodology, we were able to construct emotional arcs that depict the fluctuations in different emotions along the sequences of the text. Figure 1 presents the outcomes of our computations, illustrating the emotional arcs derived from all 66 stories.
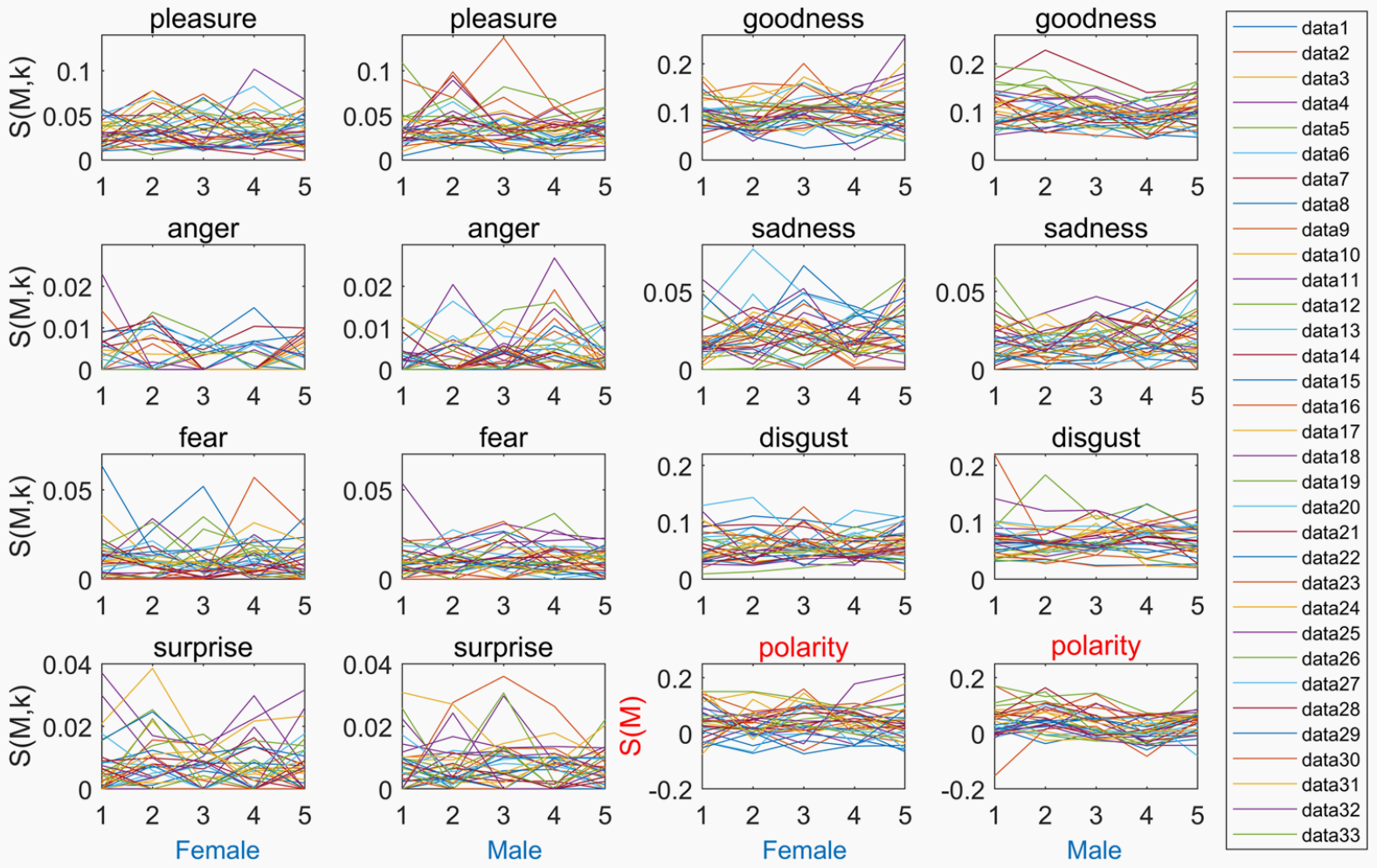
Fig. 1 The emotional arc of 66 science fiction stories. The horizontal axis of 1–5 corresponds to the 5 text sequences of each work, and the vertical axis isthe emotion value of the corresponding sequence. Each of the two columns corresponds to an emotion that is labeled at the top, where female works areon the left and male works on the right. The last two graphs show the emotion polarity values. The data numbers in the legend correspond to the serialnumbers of the stories in Table 2.
To facilitate comparisons, we have juxtaposed the images of male and female writers for each emotion arc, employing the same axis range. By examining the range of the vertical axes, we observe substantial variations in the values of different emotions. Specifically, “goodness” and “disgust” exhibit the highest values, while “anger” and “surprise” demonstrate the lowest values. This discrepancy in emotion values can be attributed, in part, to variances in the vocabulary of emotion analogs within the emotion lexicon. Furthermore, we find no significant numerical disparities between the arcs of male and female writers within each emotion category.
Indeed, considering that the emotional value of each work is closely connected to its content, the trends observed in the 33 emotional arcs in each subfigure of Fig. 1 display noticeable discrepancies, making it difficult to draw direct comparisons regarding gender differences. To evaluate if there is a universal distinction between the works of male and female writers, we took the step of averaging the emotional arcs of works by writers of different genders. The results are presented in Fig. 2.
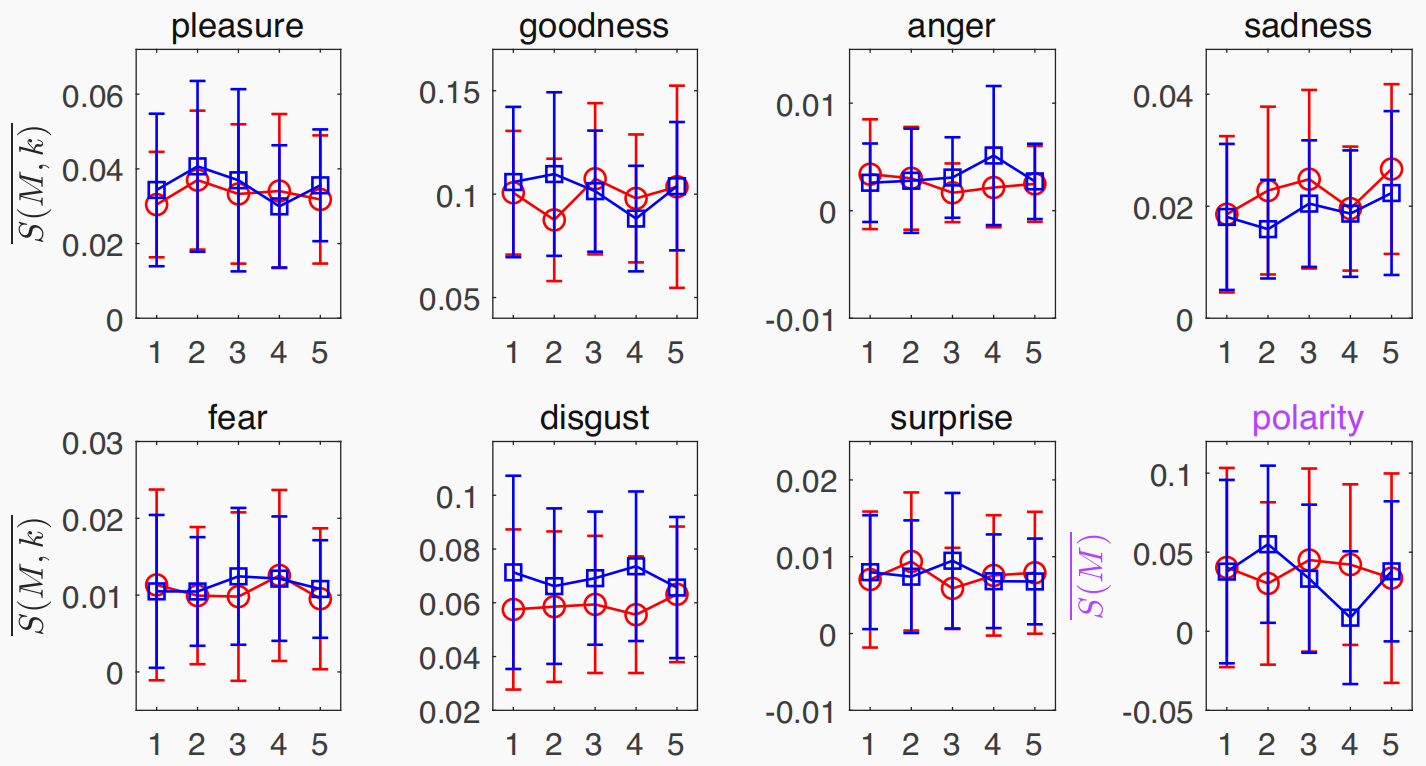
Fig. 2 Average emotional arcs for male and female works. Blue squares indicate male entries, red circles indicate female entries and vertical lines are errorbars, which are the standard deviations of the corresponding datasets. The first 7 figures correspond to the 7 different emotions, and the last figurecorresponds to the emotion polarity values.
As observed in Fig. 2, there are slight variations between male and female works in terms of the trend towards the mean. However, these differences fall within the range defined by the error bars, indicating that there is no significant distinction between the emotional arcs of male and female works in terms of statistical significance. Nevertheless, it is important to acknowledge that averaging the emotional arcs may obscure more nuanced information. Therefore, to assess the differences in emotional narratives among different works, it becomes necessary to incorporate more detailed feature quantities.
Richness and twistiness. By incorporating the two metrics of Richness and Twistiness, we can establish a two-dimensional coordinate system that captures and represents the emotional narrative qualities portrayed within the literary works. Through the calculation of coordinate points for each novel, we can discern the distribution characteristics of these works within the system. The results are shown in Fig. 3.
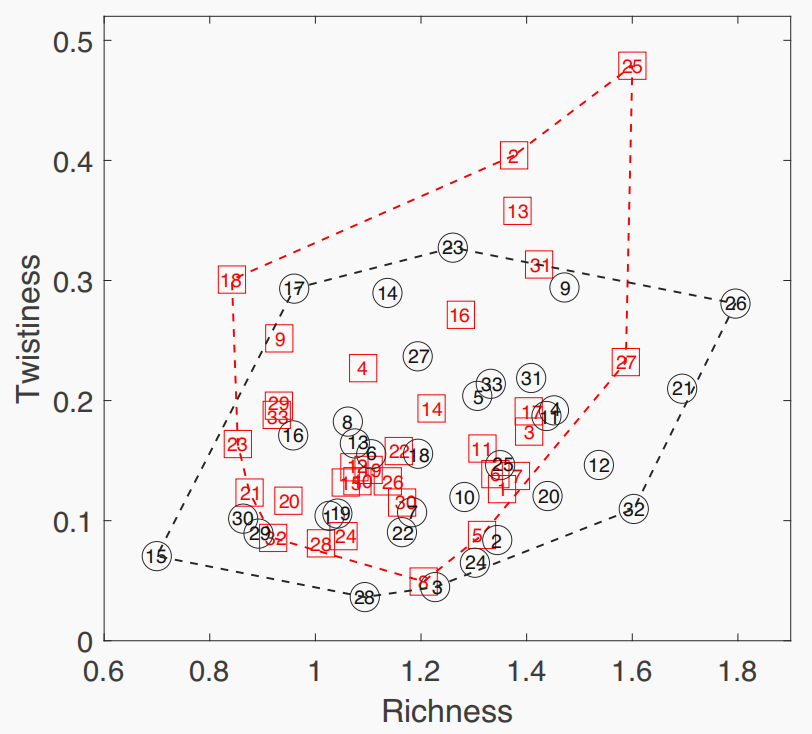
Fig. 3 Distribution of male and female works in the emotional narrativecoordinate system. The red squares represent female works and the blackcircles represent male works. The positions of the markers correspond tothe values of richness and twistiness calculated for each story, and thenumbers in the markers correspond to their numbers in Table 2. Thedashed line is the boundary line of a particular type of scatter (with acontraction factor of 0.1), which briefly frames the distribution of the twotypes of data points in the coordinate system.
The observed distribution of male and female works within the coordinate system displays a notable intermingling, lacking distinct boundaries between the two groups. The contour lines encompassing the respective ranges of both genders largely overlap. This alignment is consistent with the findings presented in Fig.
In contrast, beyond the cluster of data points, specific science fiction works exhibit distinct patterns in their emotional narratives. Notably, works in the top right corner of Fig. 3 demonstrate a heightened intensity, while those in the bottom left corner display a more subdued emotional tone. Remarkably, within these off-center points, the top right corner is predominantly occupied by female works (red 25, 2, 13), whereas the bottom left corner is primarily represented by male works (black 15, 29, 30). This observation sheds light on the perception held by certain readers or researchers that female authors tend to emphasize emotional narratives to a greater extent.
Subsequently, we select red 25, positioned nearest to the top right corner, and black 15, situated closest to the bottom left corner, as exemplary cases for further scrutiny. Through a close reading of the accompanying text, we aim to uncover the reasons behind their marginalized placement in the coordinate system. As evident from Table 2, these two works are Yin Kemi’s “No Matter What’s Real” and Liu Cixin’s “Take Her Eyes”. We extract their respective emotional arcs from Fig. 1 and represent them individually in Fig. 4.
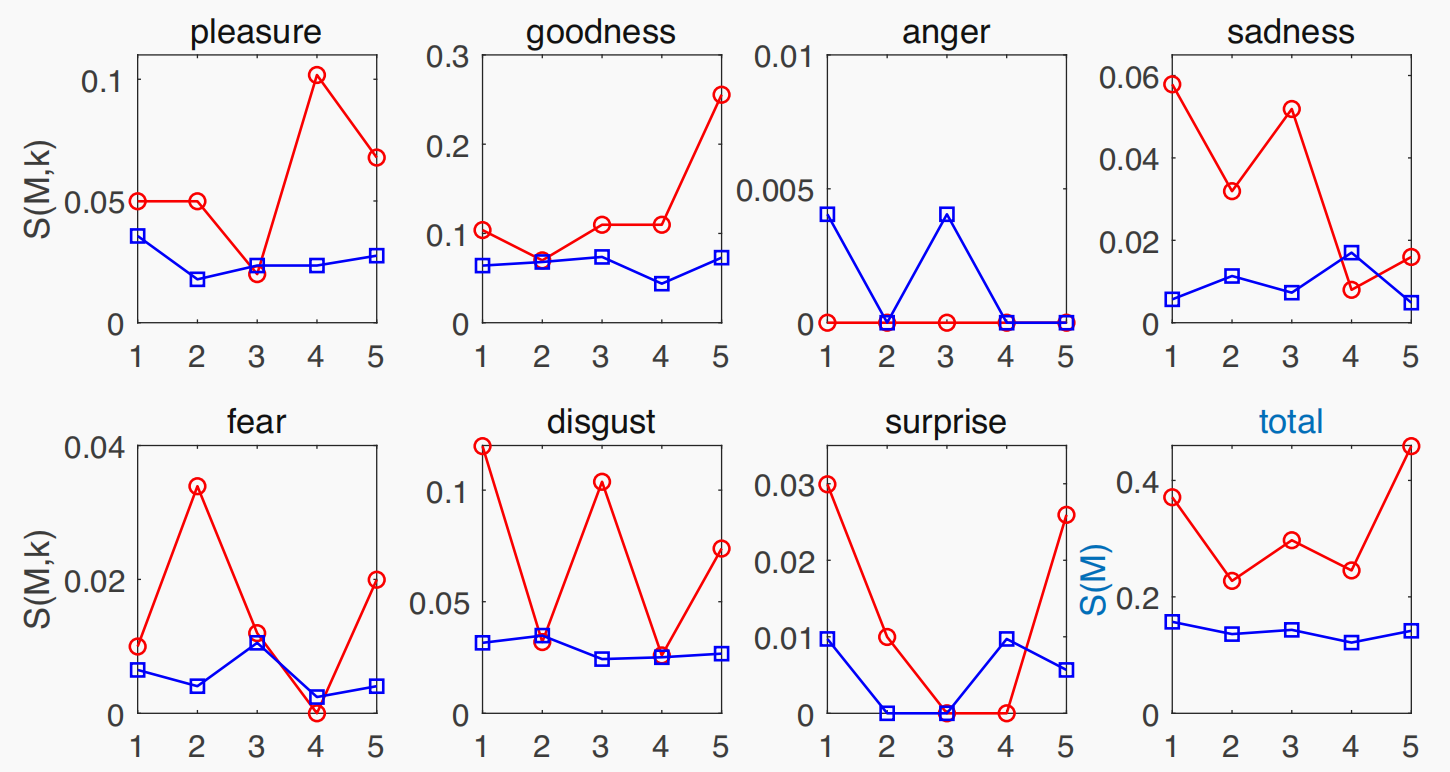
Fig. 4 Comparison of emotional arcs for two specific texts. The red circle corresponds to “No Matter What’s Real” and the blue square corresponds to“Take Her Eyes”. The last figure shows the result of adding up all the emotions.
It is evident that, in the majority of sequences and emotions, “No Matter What’s Real” exhibits higher intensity compared to “Take Her Eyes”. Additionally, the former displays a greater magnitude of emotional fluctuations. These factors contribute to the greater Richness and Twistiness of the former compared to the latter. Through careful examination of the text, we can truly appreciate the literary mechanisms underlying these data and images.
In the initial section of “No Matter What’s Real”, the protagonist undergoes a thrilling near-death experience, only to encounter accidental amnesia upon resurrection. This results in the elicitation of emotions such as “disgust”, “ sadness” and “ surprise”, which exhibit high emotional values. In the subsequent section, an unknown man enters the protagonist’s residence claiming to be her boyfriend, a claim corroborated by her best friend. However, she lacks any memory of this man and becomes terror-stricken, struggling to navigate the relationship. This leads to a sharp rise in the emotion of “fear” in this segment. In the third section, she visits the company responsible for the aforementioned experience in an attempt to retrieve her memories. Despite even threatening legal recourse, her efforts prove futile, resulting in the resurgence of negative emotions like “disgust” and “sadness”. In the fourth part, her boyfriend resolves to pursue her once again, leading to a rediscovery of their previous sweetness and eventual marriage. During this phase, the emotion of “pleasure” reaches its pinnacle, while negative emotions such as “fear,” “disgust,” and “sadness” are minimized. In the final section, on their tenth wedding anniversary, the husband confesses that the memory loss was a fabrication orchestrated by her friends. This revelation prompts a reversal of the main character’s emotions. It can be observed that considerable emotional fluctuations occur between each part of this novel, which is the reason for its high degree of Twistiness.
Notably, Yin Kemi tends to explicitly depict the protagonist’s inner feelings, exemplified by statements such as “Yan had no intention of blaming or complaining about me, which made me feel even more guilty…. Sometimes we said a few inconsequential words, and then all of a sudden, we fell into an awkward silence. The look on his face at that time … was heartbreaking.” This style of portrayal introduces more emotional words, leading to an increase in emotional Richness.
Contrasting with the emotionally vivid narrative of “No Matter What’s Real”, the novel “Take Her Eyes” adopts a restrained approach. While the core of the story conveys an extreme tragedy, the narrative perspective intentionally maintains distance from the tragic protagonist—a young girl—and instead follows an ordinary man who is able to perceive the world through the girl’s eyes. Throughout the story’s progression, there are fewer direct emotional descriptions, substituted instead with extensive depictions of scenes and backgrounds, particularly in the latter half. Consequently, the overall emotional value and fluctuations within the various sections of the story are relatively modest.
Observations of key writers. Using the aforementioned approach, we conducted calculations to determine the emotional richness and twistiness of all works within the corpus of the key writers. These values were then plotted on the same coordinate system as Fig. 3. In order to ensure comparability, we maintained a consistent range of axes across all coordinate systems.
Figure 5 displays the distribution of works by the 12 key science fiction writers on the emotional narrative coordinate system. Notably, there is a noticeable disparity in terms of the central position and extent of extension across different images in the two coordinate dimensions. For instance, when comparing Liu Cixin’s position to that of Wang Jinkang, it is evident that Liu Cixin’s works exhibit a significantly more leftward central position, indicating a lower overall emotional richness. This suggests that Liu Cixin adopts a more restrained emotional narrative approach in his works. In fact, Liu Cixin’s stories exemplify hard-core science fiction, consistently featuring a technological backdrop with the characters occupying a secondary role. Another science fiction writer He Xi once commented that Liu Cixin was “an indifferent observer of the universe, a dispassionate moral arbiter, and a contemplative thinker.” (Huang, 2011) Regarding emotional cues, he “rarely places the romantic relationships at the forefront of his emotional exploration,”(Wu and Fang, 2006, p. 38) instead delving further into the dynamics between fathers and sons in his works, contributing to the perceived cool and composed emotional narratives in his works. On the other hand, Wang Jinkang’s science fiction typically revolves around the characters, as evidenced in his “New Human” series, which explores the living conditions and emotional states of mutated individuals in the context of genetic modification and parasitic alien species. Additionally, love is a common emotional element in Wang Jinkang’s stories. These factors significantly elevate the emotional richness of his works in comparison to Liu Cixin.
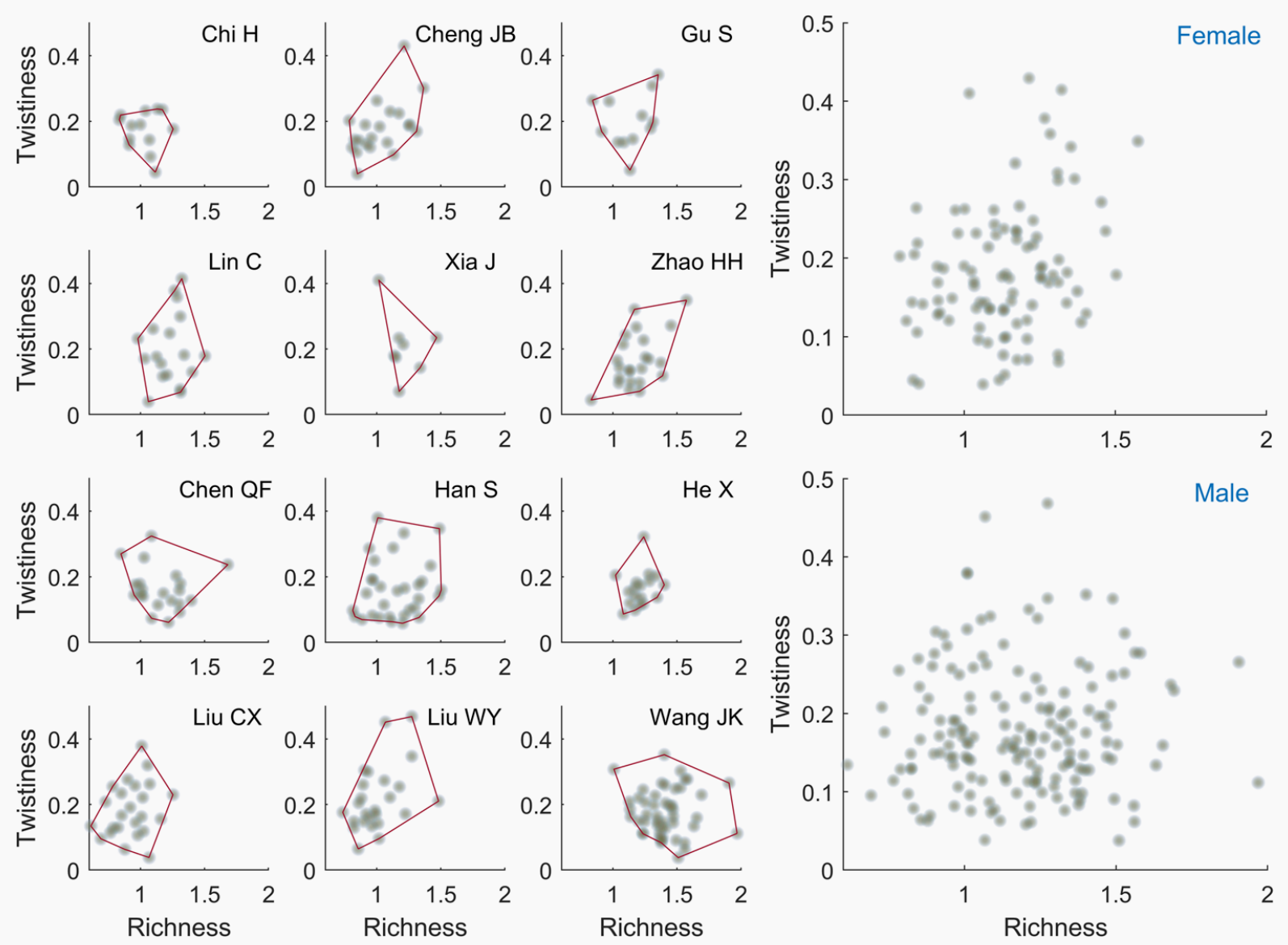
Fig. 5 Distribution of the works of the 12 key writers in the emotional narrative coordinate system. The initial two rows signify works authored by femalewriters, while the subsequent two rows pertain to male writers. The abbreviated form of each writer’s name is exhibited in the upper right corner of therespective subplot. The boundary of each scatterplot is illustrated by a solid red line. The expansive scatter plots on the right-hand side exhibit thecombined works of writers from each gender.
Furthermore, variations in clustering are discernible among the distributions. For example, both Lin Chen and He Xi have 18 works in the corpus, but Lin Chen’s distribution displays far greater dispersion. This implies that She employs a wider range of emotional narrative styles, while He Xi’s narrative styles are more uniform across different works. Lin Chen’s science fiction draws from a wide range of subjects and cover a variety of themes, and the versatility of her emotional narrative style has been noted (Zhang and Wang, 2012). For example, in “The Sky of Flying Birds”, the first-person narrative with a female character is emotionally charged, depicting various poetic scenes, while in “The Messenger”, her emotional expression is quite restrained, pursuing a lyrical tone of subtlety. In stark contrast, Chi Hui, located in the upper left corner of Fig. 5, exhibits significantly less emotional richness and twistiness in her work compared to other science fiction authors. In an interview, she expressed that science fiction is a relatively neutral literary genre and she does not wish to be defined solely as a female writer. Additionally, she acknowledged that she is “not particularly sensitive in emotional aspects, almost to the point of being dull” (Chen, 2007, p. 13). The protagonists in her works are typically male, and romantic elements are seldom involved.
However, although variations in emotional narrative styles between different writers are evident, these disparities diminish when examining the scatter plots of works categorized by the writers’ genders on the far right of the figure. Specifically, there is an equivalent degree of variation between writers within each gender group as there is between two writers of different genders. In order to provide a clearer perspective, we introduce a quantitative concept, termed “similarity”, which is based on the boundary line of each writer’s work distribution in Fig. 5. Similarity is defined by the following equation:
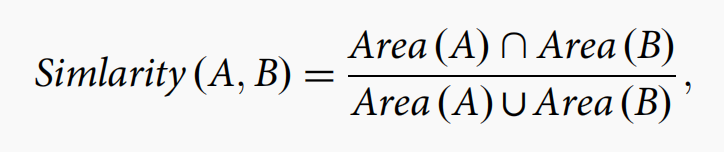
where Area (A) represents the area enclosed by the scatterplot boundary line of writer A. The numerator denotes the area of intersection between the boundary of writers A and B, while the denominator represents the combined range of the two boundaries. Notably, a writer’s similarity to themselves is naturally 1, while a similarity of 0 indicates no overlapping region between the boundaries of the two writers. In general, similarity values fall within the interval of (0,1). Figure 6 illustrates the outcomes obtained from computing the similarity between all writers.
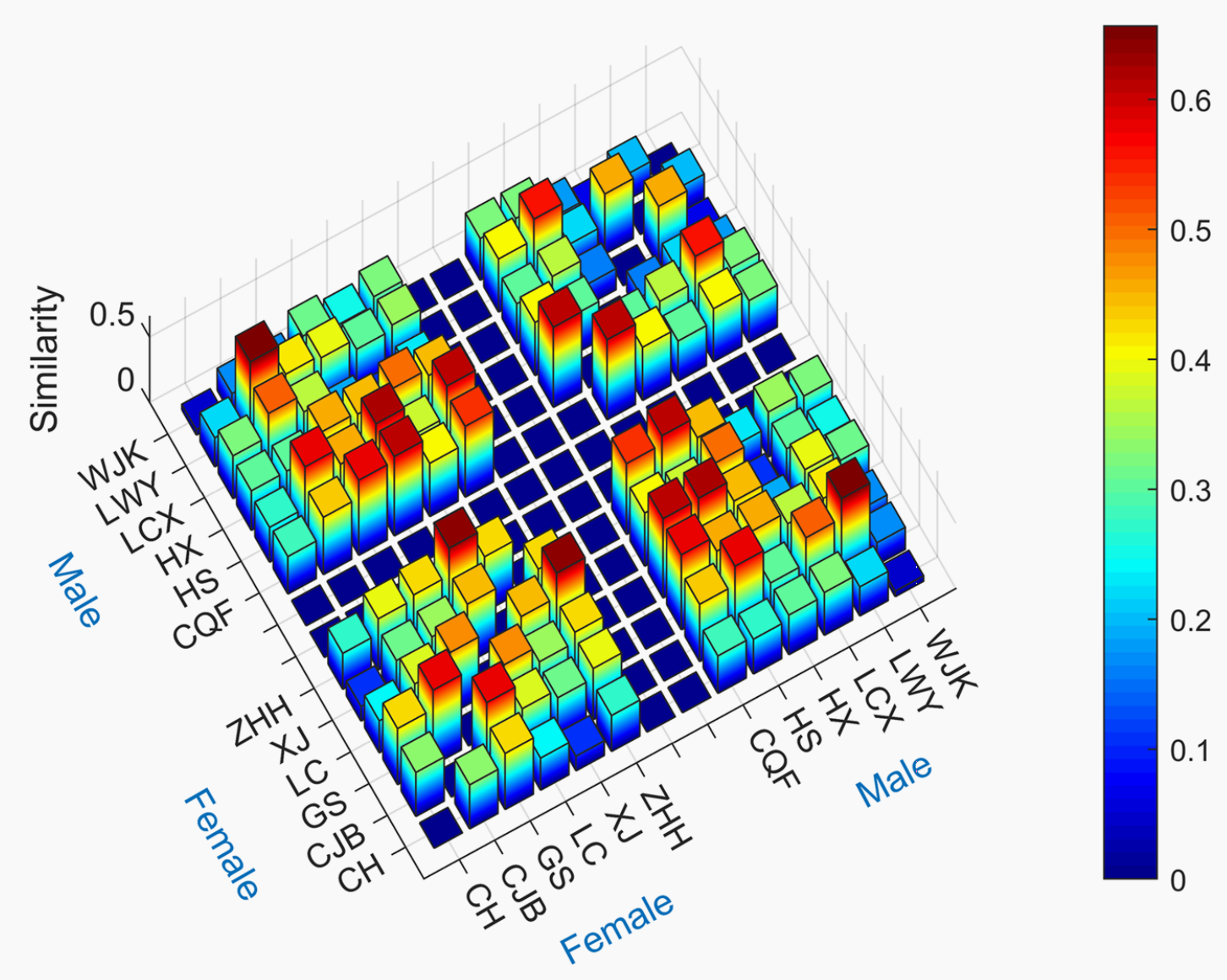
Fig. 6 Similarity of the 12 key writers in their emotional narratives. In order to facilitate observation and comparison, the similarity values are representedby the height and color of the bars. The horizontal and vertical axes of the graph are labeled with the abbreviated names of the writers for correspondingpositions. It is important to note that the similarity values between the writers and themselves are not depicted in the graph.
In the graph, we have excluded the writer’s similarity to themselves as it would dominate the bar graph, potentially obstructing the visibility of other features and lacking meaningful interpretation on its own. Additionally, we have introduced a distinct gap between male and female writers to facilitate observation and analysis. This partition divides the histogram into four distinct regions, each containing positions characterized by both high and low similarity values.
Interestingly, for the 12 writers depicted in this graph, the number of bars with high similarity values in the male-female comparison region is proportionally higher than the number of tall bars in the same gender’s region. This finding reinforces our previous conclusion that the differences between writers of the same gender are not any less pronounced than those between writers of different genders. Consequently, when discussing variations in emotional narratives, it is essential to recognize that these distinctions result from the authors’ unique creative styles, rather than from disparities in their genders.
Discussion
In the conventional practice of literature analysis and study, individuals often engage with a limited selection of works, potentially leading to erroneous generalizations wherein the qualities of a few works are misattributed as defining characteristics of an entire genre or category. For instance, if we were to solely read and interpret a few notable works by authors such as Liu Cixin, Yin Kemi, and Zhao Haihong, it is highly probable that we might erroneously conclude that female authors primarily focus on the portrayal of emotions. This situation highlights the distinct advantage offered by the emerging “digital humanities” trend in recent years. Moretti has offered a critique of the traditional approach to the study of world literature, contending that the scope of the field is constrained to the analysis of a small number of classical texts, leading to substantial skepticism regarding the “conceptual cogency that a small set of texts allows for” (Moretti, 2013, p. 2). Significantly, our study serves as a tangible exposition of this view, as corpus-based research shows that there is no stylistic difference in emotional narratives between science fiction writers of different genders, contrary to some traditional beliefs.
The genesis of the above findings can be understood in three ways. First, whether in male or female writers, the main perspective of the narrative predominantly features male characters. Among the 66 works presented in Table 2, there are 10 works by female writers and 7 works by male writers with a predominantly female perspective. Although the former slightly outweighs the latter, the overall percentage remains very low. This choice is partly driven by narrative necessity but often reflects cultural inertia, such as the prevalent portrayal of scientists as male in almost all works. Consequently, when female writers depict a male character, their emotional empathy and sensitivity naturally tend to be weaker. Secondly, before the 1980s, Chinese science fiction had a strong emphasis on science popularization function and primarily targeted teenage readers (Wu, 2013). While this function has diminished since then, many works still exhibit a children’s literature style. In China, the number of adult science fiction works published annually is comparable to that of children’s science fiction works (Cui and Zhu, 2023). In addition to authors specializing in children’s science fiction, many adult science fiction authors, such as Wang Jinkang and Jiang Bo, often publish science fiction works intended for children. In the corpus analyzed, some works, such as “Nature Lesson of Class 4.1” and “ Tongtong’s Summer”, that fall into the category of children’s science fiction. In these works, the characters’ emotions are typically simpler and more direct, with fewer twists and turns. Thirdly, in many science fiction works, the emotions of the characters are not the primary focus for writers and readers. Instead, the narrative’s core lies in the presentation of the science fiction setting and the evocation of a sense of wonder. In some works, the character serves as a purely functional entity, and the exploration of their inner world often takes a secondary position. Consequently, in such works, emotion becomes a marginal presence in the narrative process. Similar to male writers, many female science fiction writers also adopt such a creative approach. These factors have collectively contributed to the suppression of stylistic differences in the emotional narratives of male and female science fiction writers, resulting in an overall convergence.
Acknowledging the existing limitations of this study is essential. One notable concern involves the method employed to truncate the text based on an average word count, as previously mentioned. This approach may result in the fragmentation of context and the inadvertent division of the same narrative scene into multiple chronological segments, potentially impacting the reliability of the effective measure. In an ideal scenario, the work would be segregated based on its narrative scenes to ensure the preservation of the integrity and independence of affective values within each sequence. Nonetheless, while there have been initial forays into automating the slicing of texts according to narrative scenes (Kozima and Furugori, 1994), the algorithm in this area is still far from being fully developed.
Some limitations arise from the localized failures of sentiment computing algorithms when confronted with the stylistic diversity and narrative complexity found in literary works. Authors may opt to convey a scene not explicitly but through indirect descriptions that imply events or evoke a particular mood. Unfortunately, emotional calculations lack sensitivity towards this type of writing. Similar shortcomings occur when sarcasm and deception are implied in character dialog.
Moreover, contemporary sentiment calculations commonly rely on isolated units of short texts, such as words and sentences, to generate quantitative sentiment values. Consequently, elements like the story background, previous text buildup, and character development are easily overlooked, thereby introducing potential bias into the calculations. In other words, the apparent and conventional polarity and emotional categories associated with emotion words in literary works may not necessarily apply within a specific context. Reed (2018) explored this issue by analyzing poetry from the Black Arts Movement in the United States, investigating how emotions related to injustice were encoded based on race and gender using affective calculation. However, he discovered a disconnect between the surface meanings of emotional words and their more nuanced, deeper emotions shaped by textual, social, and political contexts. Enhancing the accuracy and validity of sentiment recognition remains a major challenge for ongoing research in affective computing.
Data availability
The datasets analyzed during the current study are available in the Dataverse repository: https://doi.org/10.7910/DVN/P0THSF.
References
Anderson CW, McMaster GE (1982) Computer assisted modeling of affective tone in written documents. Comput Hum 16:1–9. https://doi.org/10.1007/BF02259727
Barros L, Rodriguez P, Ortigosa A (2013) Automatic classification of literature pieces by emotion detection: a study on Quevedo’s poetry. In: 2013 Humaine Association Conference on Affective Computing and Intelligent Interaction. IEEE, pp 141–146
Chen JR (2022) Emotional processing and evaluation in translation from the perspective of affective narratology: a case study on the English translation of the feminine literary work maidenhome. Foreign Lang Their Teach 3:94–102
Chen QF (2007) Finding your own story—an interview with Chi Hui, a new science fiction author. World Sci Fiction Expo 10:13–14
Cui XP, Zhu R (2023) The creative landscape and reflections on Chinese children’s science fiction in 2022. Sci Writ Rev 3(03):12–19
Ekman P (1992) An argument for basic emotions. Cogn Emot 6(3-4):169–200
Elkins K, Chun J (2019) Can sentiment analysis reveal structure in a plotless novel? https://arxiv.org/abs/1910.01441
Elsner M (2015) Abstract representations of plot structure. Linguist Issue Lang Technol. 12. https://doi.org/10.33011/lilt.v12i.1381
Grysman A, Fivush R, Merrill NA et al. (2016) The influence of gender and gender typicality on autobiographical memory across event types and age groups. Mem Cogn 44:856–868
Huang YM (2011) Every civilization is a hunter with a gun. Southern Weekend, April 26. https://www.infzm.com/contents/58004?source=131
Kim E, Klinger R (2018) Who feels what and why? Annotation of a literature corpus with semantic roles of emotions. In: Proceedings of the 27th International Conference on Computational Linguistics. Association for Computational Linguistics, Santa Fe, New Mexico, USA, pp 1345–1359
Kim E, Klinger R (2021) A survey on sentiment and emotion analysis for computational literary studies. Zeitschrift Für Digitale Geisteswissenschaften Wolfenbüttel. https://doi.org/10.17175/2019_008_v2
Koppel M, Argamon S, Shimoni AR (2002) Automatically categorizing written texts by author gender. Lit Linguist Comput 17(4):401–412
Kozima H, Furugori T (1994) Segmenting narrative text into coherent scenes. Lit Linguist Comput 9(1):13–19
Lettieri G, Handjaras G, Bucci E et al. (2023) How male and female literary authors write about affect across cultures and over historical periods. Affec Sci. 4:770–780
Mendenhall TC (1887) The characteristic curves of composition. Science 9(214, supplement):237–249
Moretti F (2013) Modern European literature: a geographical sketch. In: Distant reading, Verso Books. pp 1–42
Reagan AJ, Mitchell L, Kiley D et al. (2016) The emotional arcs of stories are dominated by six basic shapes. EPJ Data Sci 5(1):1–12. https://doi.org/10. 1140/epjds/s13688-016-0093-1
Reed E (2018) Measured unrest in the poetry of the black arts movement. In: Digital Humanities 2018: Book of Abstracts. Hg. von Jonathan Girón Palau / Isabel Galina Russell, pp 477–478
Samothrakis S, Fasli M (2015) Emotional sentence annotation helps predict fiction genre. PLoS ONE 10(11):e0141922. https://doi.org/10.1371/journal.pone.0141922
Schmidt T, Burghardt M, Dennerlein K (2018) Sentiment annotation of historic German plays: an empirical study on annotation behavior. In: Proceedings of the Workshop on Annotation in Digital Humanities 2018. RWTH Aachen, Sofia, Bulgaria, pp 47–52
Song MW (2022) The gender question in science fiction: transcending binary poetics of imagination. Shanghai Lit 5:126–136
Wang SY (2022) Exploring the publication strategy of Chinese women science fiction writers’ anthologies. Jinggu Cult Creat Ind 32:25–27
Wu Y (2011) Outline of science fiction. Chongqing Publishing House
Wu Y, Fang XQ (2006) Liu Cixin and neo-classical science fiction. J Hunan Univ Sci Eng 2:36–39
Wu Y (2013) “Great Wall Planet”: introducing Chinese science fiction. Sci Fiction Stud 40(1):1–14
Xu LH, Lin HF, Pan Y, Ren H, Chen JM (2008) Constructing the affective lexicon ontology. J China Soc Sci Tech Inf 27(2):180–185
Yao HJ, Zhao HH, Cheng JB (2021) Three perspectives on Chinese Women’s science fiction. In: She: classic works collection of Chinese women science fiction writers. China Radio and Television Publishing House
Zhang YH, Wang WY (2012) Mutual reflections of realism and romanticism: Ling Chen’s Science Fiction World. Humanit Soc Sci J Hainan Univ30(5):54–58